UMD Research Scientist Unveils New Strategies to Search Large DNA Databases
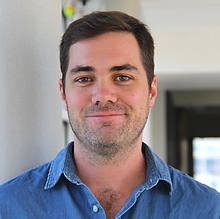
Sometimes bigger is not necessarily better. Take DNA databases, for example. As a result of substantial advances in computing power, scientists can now rapidly slice and dice genomic data used to identify different species of bacteria, or at least find their close relatives.
This is relevant for researchers wanting to match unknown DNA sequences against a database of known sequences, helping to answer fundamental biological questions such as what organism is this DNA from, or what gene might it encode?
But DNA databases used in these searches have grown so large, so quickly, sometimes undermining the scientific process. This may cause medical or environmental researchers to conclude that their as-yet-unidentified samples contain potentially harmful organisms, when in fact they don’t.
A research scientist in the University of Maryland’s Center for Bioinformatics and Computational Biology (CBCB) has just published an analysis that demonstrates a call for improved methods and algorithms when searching large DNA databases.
Daniel Nasko (in photo), working with experts from Rice University and the National Human Genome Research Institute, detailed current challenges and offered several strategies to improve the accuracy of DNA classifications using high-throughput sequencing tools. The goal is to rapidly classify microbes more confidently.
The research team’s analysis recently appeared in Genome Biology. Nasko was lead author of the study. Todd Treangen (lead researcher on the team from Rice University), Sergey Koren and Adam Phillippy (both from the National Human Genome Research Institute) also contributed.
“This work should highlight that more information does not automatically lead to more knowledge,” says Nasko, who has an appointment in the University of Maryland Institute for Advanced Computer Studies (UMIACS). “By sequencing so many similar bacterial genomes, we’re going to need more advanced algorithms and metrics to determine the biological origin of pieces of DNA.”
For their study, the researchers closely examined RefSeq, a widely used federal database that stores genomes from all of the organisms that have been sequenced. RefSeq currently contains about 1.3 trillion base pairs of data (>1.3 petabytes of data), which makes searching against this database computationally challenging.
The researchers tracked how the addition of more bacterial genomes to the database has made it more difficult for fast algorithms to identify what bacterial species an unknown piece of DNA originated from.
This may be happening because new species of common bacteria are being sequenced over and over again, they say. For example, more than five percent of the bacterial genomes in RefSeq belong to Pseudomonas bacteria (Pseudomonas is only one of nearly 3,300 bacterial genera in RefSeq).
A primary concern for Treangen, an assistant professor of computer science at Rice University who is an expert in the study of genetic material from environmental samples, is maintaining the ability to quickly identify bacteria that pose a threat to public health.
Big data is uniquely positioned to do this, Treangen says, but there’s just so much of it. At present, he explains, low-cost and high-throughput DNA shotgun sequencing machines—which read short DNA sequences from collections of microorganisms—have resulted in the doubling of genomic data in RefSeq every two to three years.
“I initially thought more data is always better for these methods,” says Treangen, who worked closely with Nasko at UMIACS for several years before relocating to Rice earlier this year. “You would expect that there would be no penalty, because database growth is good.”
However, the researchers found that bacterial data in RefSeq has an outsized effect at the species level of the taxonomic hierarchy, which is growing at a breakneck pace.
That’s a problem for scientists who combine two common techniques to identify what they find. One is called k-mer-based classification, which identifies short DNA sequences from all the organisms in a bacterial sample via exact matches.
Most of the methods that have made the problem computationally feasible rely on k-mers, which are exact matches of DNA strings of length ‘k,’ says Treangen. “If a sequenced read perfectly matches something in the database, the intuition is that you can say what that is with great precision and shortcut more expensive computational approaches,” he says.
A commonly used technique with k-mer-based classification is lowest common ancestor (LCA) assignment. LCA assesses all of the organisms an unknown piece of DNA matched, assigning them if necessary to a higher level in the taxonomy, such as a genus rather than a species. But this may not be specific enough for researchers trying to pin down a pathogen.
In fact, the study by Nasko and the other researchers found a k-mer-based classification tool called Bracken, which uses Bayesian statistics to infer the best match for a sequence, which helped mitigate the imbalance. Even so, it struggled to identify genomes with close relatives, but not perfect matches, in the database.
Treangen says well-funded research into particular pathogens is a necessity and has greatly aided rapid-outbreak detection and tracking, but it ultimately biases public databases like RefSeq.
“For instance, there's an immense bias toward foodborne pathogens,” he says. “Society wants to know a lot about Salmonella, and rightfully so. The FDA, and specifically GenomeTrakr, have aided in the sequencing of thousands of relevant pathogens and have added them directly to the reference database.”
However, Treangen says, that skews the reference database toward particular genera and families of microbes in a way that affects the accuracy and sensitivity of fast taxonomic-classification tools like Kraken that use k-mer and LCA-based approaches.
Nasko says the best recent example of a false positive identification is a study that initially reported evidence of deadly bacteria in New York City’s subways. The study, based on sequenced genomes from samples, was later revised to reflect mismatches that falsely identified the sequences of Bacillus cereus (a common bacterium not harmful to humans) as Bacillus anthracis (the bacteria responsible for anthrax).
While a focus on public health is a key priority, the research team says novel techniques able to cope with database growth and noise, coupled with an increased breadth of sequenced genomes, is needed for continued improvements in the field.
“The genetic boundaries that once clearly divided certain bacteria from others are beginning to get a bit murkier as we continue to see more regions different genomes that are shared across species and genera of microbes,” Nasko says. “Our analysis demonstrates that current computational tools make searches against large databases possible, and that’s important, but more sensitive searching strategies are still needed to improve the accuracy of these DNA classifications.”
###
The research was supported by the Division of Intramural Research of the National Human Genome Research Institute, part of the National Institutes of Health, and the Intelligence Advanced Research Projects Activity via the Army Research Office.
This article was adapted from a news release published by Rice University.